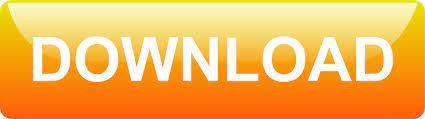
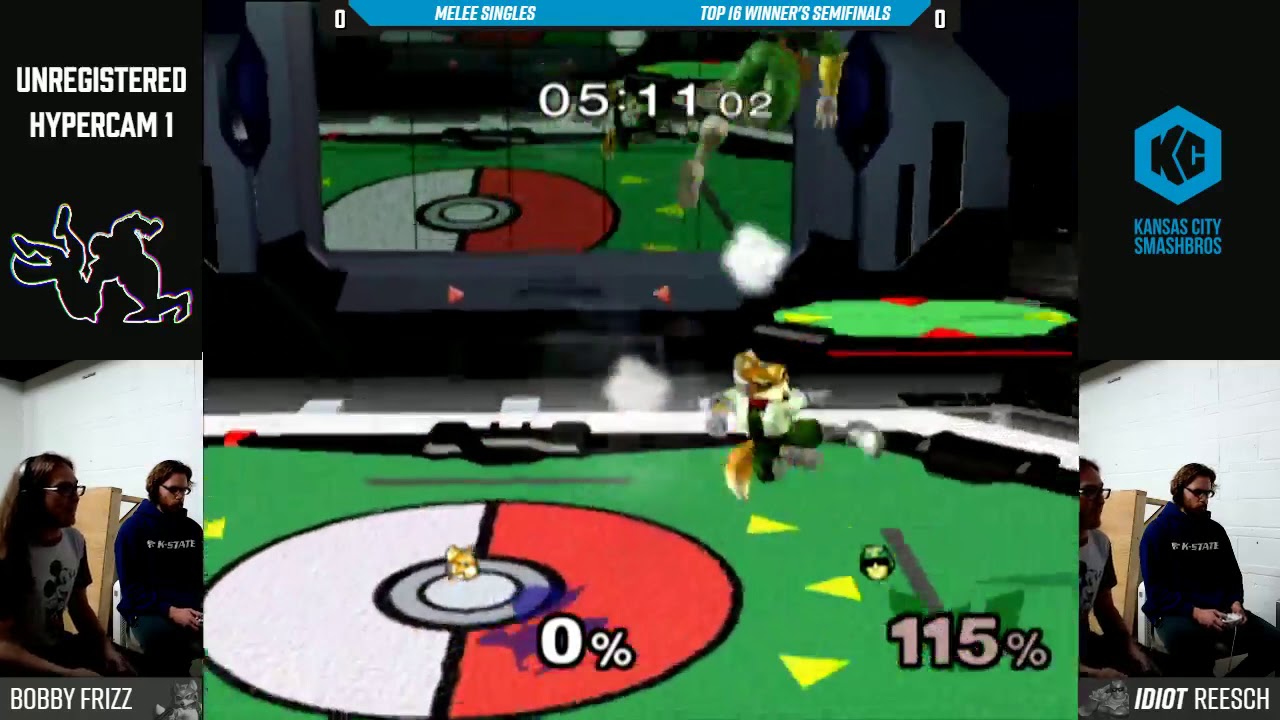
The report reviewed current systems for monitoring gas leaks at industrial facilities. In addition, the ablation study with respect to different combinations of the proposed structure including direct classification and unmixing methods has revealed the contribution of the proposed system. The superior performance of the proposed method with respect to the conventional hyperspectral gas detection methods using spectral angle mapper (SAM) and adaptive cosine estimator (ACE) is verified with LWIR hyperspectral images including Methane and Sulphur Dioxide gases. Finally, the detection is achieved by a three-layer fully connected network to detect the target gases at each pixel based on the extracted endmember spectra and abundance values. Then, a 3D CNN and autoencoder-based network, which is specially designed for unmixing, is applied to the resulting data to acquire abundances and endmembers for each pixel.

The proposed method first converts the radiance data to luminance-temperature data. Observing that the existing hyperspectral gas detection methods in the thermal range neglect the fact that the captured radiance the in longwave infrared (LWIR) spectrum is better modeled as a mixture of the radiance of background and target gases, we propose a deep learning-based hyperspectral gas detection method in this paper, which combines unmixing and classification. Hyperspectral image analysis offers many advantages over traditional gas detection systems with its detection capability from safe distances. The detection of gas emission levels is a crucial problem for ecology and human health.
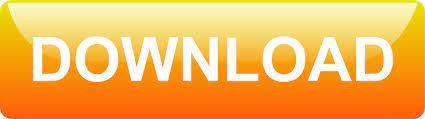